Boon Logic’s Unsupervised Learning algorithm, Nano, Performs 1,000 Times Faster than Traditional Algorithms Unlocking Massive Problem-Solving Potential Across Every Sector
Boon Logic’s Unsupervised Learning algorithm, Nano, Performs 1,000 Times Faster than Traditional Algorithms Unlocking Massive Problem-Solving Potential Across Every Sector
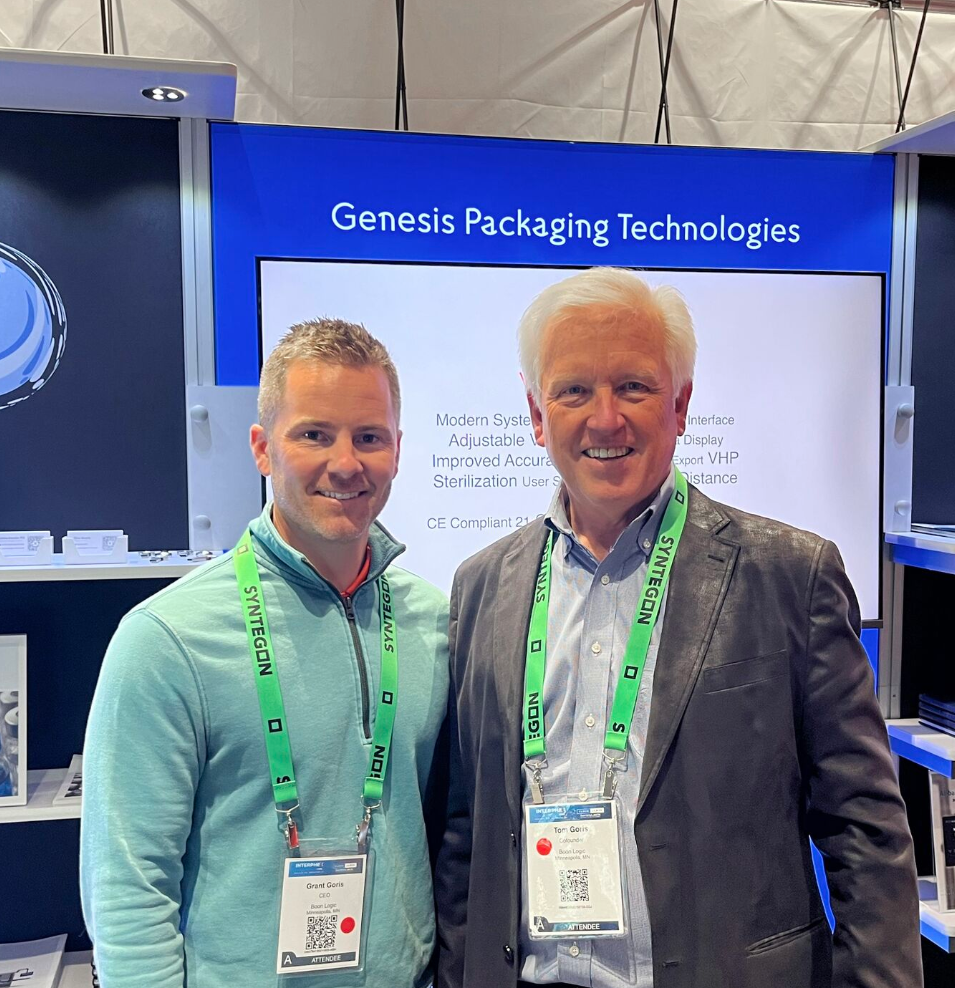
In the world of machine learning, clustering or cluster analysis is used to group data points and multi-variate feature vectors based on their similarity with each other. This method, defined under the branch of Unsupervised Learning, aims at gaining insights from unlabelled data points. Clustering algorithms are commonly used by large companies and in academia for market segmentation, market basket analysis, social network analysis, medical imaging, anomaly detection -- any use case where one needs to work with and analyze large datasets which are too complex or too large to assign labels to at scale or where the similarities aren’t intuitively identifiable by humans, such as is the case in signal analysis applications.
Traditionally, experts have relied on the k-means, recognized widely as one of the simplest and most popular clustering algorithms.
However, relying on k-means comes at a high cost. The algorithms require large amounts of CPU or GPU to run, multiple iterations of model training to refine, often require the pre-determination of the number of clusters or groups you are looking for.
They are also limited to a relatively small number of clusters. Leveraging massive data sets in the cloud puts traditional clustering approaches well beyond the reach of many companies.
Training models using k-means is very slow, taking weeks or even months to perform. And, k-means is also a highly sophisticated and complex algorithm that requires the specialized academic knowledge of data scientists and mathematicians. As such, clustering algorithms continue to be leveraged mostly in academia and large technology companies the likes of Google.
What if there was a simpler way?
Boon Logic, like most startups, began with a question that challenged the status quo. Its founders wondered, when it comes to segmentation and unsupervised learning, “what if there was a better, simpler, more computationally efficient way?”
“k-means has been relied upon for the last 40-50 years. Little has changed in that time” says Tom Goris, co-founder and executive chairman of Boon Logic. “But it is not a scalable or user-friendly approach.”
Through trial and error, Boon’s founders had discovered a better way. The company’s clustering technology, Nano for unsupervised machine learning, performs anomaly detection, model training and clustering 1,000 times faster than traditional modelling methods, all while leveraging a single CPU.
No one believed it was possible at first.
Says Tom, “Back eight years ago when we started Boon, the world of AI and machine learning was just emerging in the supervised realm of neural nets, also known as deep learning. GPU was just starting to emerge as the preferred architecture for building AI models. However, we were working in the realm of unsupervised machine learning, which has some similarities but is fundamentally different. On top of it, our Nano, which is what we now call our product, replaces commonly employed algorithms. k-means is no longer relevant and one class auto-encoders and principal component analysis (PCA) are very slow to train and lack accuracy once the model is built. Academics who are used to these arrows in their quiver looked at us like we had two heads. It is as if we were telling them, “You don’t need that large engine in your car anymore. We will replace it with a tiny engine that'll make you go 1,000 times faster. Put that in your car.” And their answer was, “No, I don’t believe you.”
So Boon used academia itself to deliver the proof it needed. As a first thrust to academia’s parry, it hired two respected PhDs to perform a comprehensive study comparing the speed and accuracy of the traditional methods against that of Nano. Sure enough, the result showed Nano performed the same analysis 1,000 times faster.
Boon then hired Dr. Brian Turnquist as its CTO. A respected academic with 25+ years of research in the field of AI/ML and a visiting researcher at Johns Hopkins, Yale, and Heidelberg, Brian brought to Boon impeccable academic credentials. He was also a believer.
“When Brian first met us, we were performing analysis on a little Intel Nuc. He could see we were fast, so he gave us a dataset to test. What took him twelve minutes to run the analysis on his four-core laptop, took us three seconds on one core of the Nuc. He said, “I’m never using k-means again.”
Train on the good
Today, it takes Boon Logic’s Nano one second and one core of CPU to run that same data set. This analysis can be accomplished on one pass without the need to go back and hyper-tune parameters. Nor does the technology require sophisticated mathematical knowledge to operate.
Tom explains that in the supervised machine learning world, anomaly detection models are trained off “the bad” data. And because bad data can be extremely sparse…or sometimes not previously seen with new defects, it takes some months to train models. Boon doesn’t train off defects, it trains off “compliant.” In most modern manufacturing environments, “compliant” units are the lion’s share of the data produced.
He shares a story to illustrate the point. “I know a company that paid $6 million for a 500 /minute bottle machine. It could cost them another several million to get that machine up and running and at least two months to train the model. In our office right now, I have our software running on a machine and a college sophomore is training a recipe on a syringe for a major pharma company with 500 compliant syringes, and it will take him an hour.”
Empowering AI-powered problem-solving
Boon Logic’s go-to-market strategy leverages partners including Intel, to bring Nano to a wide range of industries from pharma and healthcare, to manufacturing, defense and heavy industry.
Says Tom, “By simplifying and accelerating AI development and deployment, particularly in the domains of visual inspection and preventive maintenance, Nano empowers existing domain specialists to harness the benefits of unsupervised AI for their organizations, reducing the demand for elusive AI experts. And its versatility shines through as it autonomously fine-tunes, trains, and operates in real-time, whether at the edge or in the cloud.”
Boon Logic was introduced to the 5G Open Innovation Lab by mutual partner Intel.
“Very quickly after talking with Jim at the Lab we saw a fit and joined as part of its Batch 9 cohort,” says Tom.
The Lab also is now partnering with Boon Logic as it shifts its focus toward monetization of the 5G network, with both entities see significant potential to integrate Nano into the Lab’s Blueberry’s private 5G network in a box as a way to leverage the network to monitor machines and devices and detect anomalous behaviors.
It may have taken time to prove its value to academia, but with the right partners at its side, and proven technology and product/market fit, Boon Logic is now entering its growth phase.
Says Tom, “There are so many problems out there that we haven’t been able to solve because we have been held back by current clustering methods. It was too hard, too complex, too slow. And companies around the globe are losing millions as a result because they haven’t had a way to perform that analysis. We’re about to show the world how to fly through it.”